Robust inference of panel data models with interactive fixed effects under long memory: A frequency domain approach
Ke Shuyao, Peter C.B. Phillips, Liangjun Su. Robust inference of panel data models with interactive fixed effects under long memory: A frequency domain approach. Journal of Econometrics. April 2024. Volume 241, Issue 2.
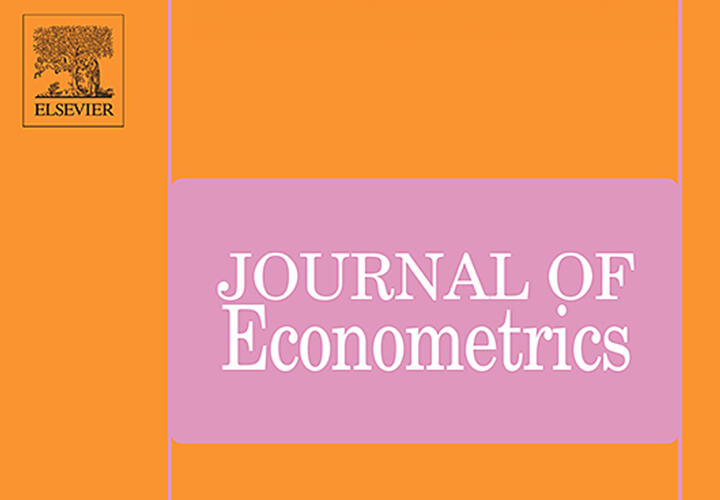
This paper studies a linear panel data model with interactive fixed effects wherein regressors, factors and idiosyncratic error terms are all stationary but with potential long memory. The setup involves a new formulation of panel data models, where weakly dependent regressors, factors and idiosyncratic errors are embedded as a special case. Standard methods based on principal component decomposition and least squares estimation, as in Bai (2009), are found to be biased and distorted in inference. To cope with this failure and to provide a simple implementable estimation procedure, a frequency domain least squares estimation is proposed. The limit distribution of the frequency domain estimator is established and a self-normalized approach to inference without the need for plug-in estimation of memory parameters is developed. Simulations show that the frequency domain estimator performs robustly under short memory and outperforms the time domain estimator when long range dependence is present. An empirical illustration is provided, examining the long-run relationship between stock returns and realized volatility.